The progression of machine learning
This content is from our sponsor. Fox Business editorial was not involved in the creation of this content.
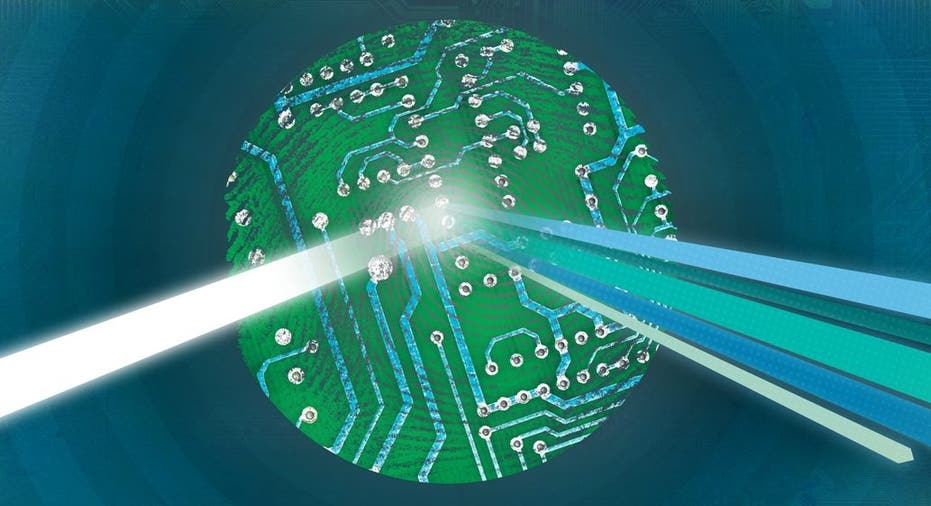
It is becoming easier, faster, and cheaper for companies of every enterprise to implement machine learning—a data-fueled artificial intelligence technology used to detect patterns and anomalies, and make predictions.
Even though industries find its capabilities appealing, most companies are not yet taking advantage of this transformative technology. As explained in “Signals for Strategists: Machine Learning and the Five Vectors of Progress,” Deloitte believes that progress in five key areas can help overcome the barriers to adoption and eventually make machine learning technology mainstream.
Obstacles of Machine Learning
According to a 2017 survey of 3100 executives in various sized companies across 17 countries, fewer than 10 percent of companies are investing in machine learning [i], despite it being considered “one of the most powerful and versatile information technologies available today.”[ii]
Deloitte points out some major factors hindering the adoption of machine learning: the short supply of qualified practitioners [iii]; the immature and still-evolving tools and frameworks for doing machine learning work [iv]; and the time-consumption and costs associated with obtaining enough data sets for machine learning model development.[v]
It’s not atypical for an emerging technology to be overly expensive to implement, but another issue impeding machine learning adoption is the reluctance of executives to deploy a tool whose inner workings they cannot understand or clearly explain, even though its adoption would prove valuable to their company.
Progress in Five Areas
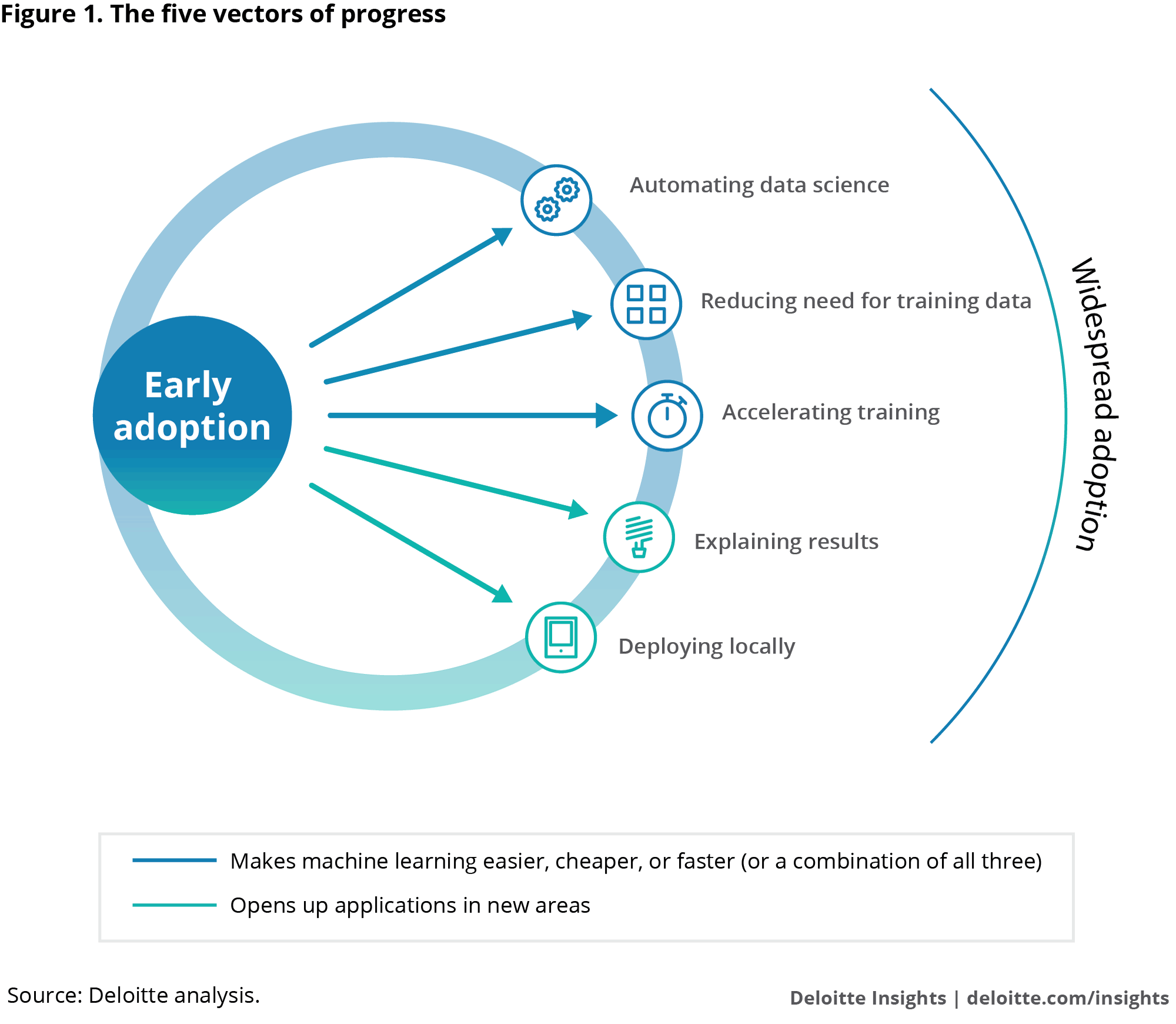
Deloitte believes that the barriers of adoption are beginning to fall and pinpoints five key vectors of progress that should help increase the deployment of machine learning. The first three make machine learning easier, cheaper, and/or faster to implement—helping ramp up usage in existing areas—while the last two will help expand machine learning applications into new areas.
Automating data science. Data science, a prerequisite for developing machine learning solutions, can be partially or fully automated, freeing up data scientists—who are in high demand but short supply—to be productive elsewhere. Instead of taking months to execute a machine learning proof of concept, automating data science could reduce that length to days.[vi]
Reducing need for training data. Acquiring and labeling the massive amount of data (potentially in the millions of elements) needed for machine learning has proven to be costly and time-consuming, making techniques that can reduce the needed amount of training data invaluable. One such technique involves using synthetic data generated by algorithms to mimic the characteristics of real data.[vii] A Deloitte team tested a tool that built an accurate model starting with only 20 percent of the typical amount of data required, and had algorithms produce the rest. Pre-training a machine learning model with one data set that can be used to learn other data sets in similar domains (think language translation or image recognition) is called transfer learning, and is another technique that could reduce the need for training data.
Accelerating training. Semiconductor and computer manufacturers are drastically reducing the amount of time required to train machine learning models by developing special processors such as graphics processing units (GPUs) to accelerate calculations and speed up the transfer of data within the chip. As the required training time shrinks, so too do the associated costs. Adoption of these specialized chips is spreading, and as every major cloud provider offers GPU cloud computing, increases in productivity through accelerated training are becoming possible.
Explaining results. Certain industries cannot use machine learning models because it’s not possible to explain how the model makes decisions. A medical diagnosis or a financial transaction will oftentimes not be accepted without the rationale to support the predicted outcome. But techniques used to see inside the black box of models are becoming available, and as it becomes possible to build interpretable machine learning models, highly regulated industries will now be able to capitalize and take advantage.
Deploying locally. Machine learning adoption will grow as it becomes possible to deploy the technology into new areas. Recent advancements in hardware and software are enabling its use on mobile devices and smart sensors [viii], significantly expanding the possibilities of use in areas like smart homes, autonomous vehicles, wearable technology, and the Internet of Things.
Click Here for More Insights from Deloitte
Authors: David Schatsky, Rameeta Chauhan
[i] SAP, “Digital transformation executive study,” 2017.
[ii] David Schatsky, Craig Muraskin, and Ragu Gurumurthy, Demystifying artificial intelligence, Deloitte University Press, November 4, 2014.
[iii] Will Markow, Soumya Braganza, Steven Miller, and Debbie Hughes, “The quant crunch: How the demand for data science skills is disrupting the job market,” IBM, Burning Glass, and BHEF, 2017.
[iv] Catherine Dong, “The evolution of machine learning,” TechCrunch, August 8, 2017.
[v] Alex Ratner, Stephen Bach, Paroma Varma, and Chris Ré, “Weak supervision: The new programming paradigm for machine learning,” Stanford Dawn, July 16, 2017.
[vi] Gregory Piatetsky, “Automated data science and data mining,” KDnuggets, March 2016.
[vii] Sergey Nikolenko, “New resources for deep learning with the Neuromation platform,” Neuromation.io, October 9, 2017.
[viii] David Schatsky, Machine learning is going mobile, Deloitte University Press, April 1, 2016.